Research Data Management: Good Scientific Practice applied to Data
A central component of GSP is good research data management (RDM).
This refers to every step in the
(image below), from generation over analysis to publishing and preservation of data.Following FAIR principles ensures that the basis and outcomes of scientific work are of high quality and sustainably useful.
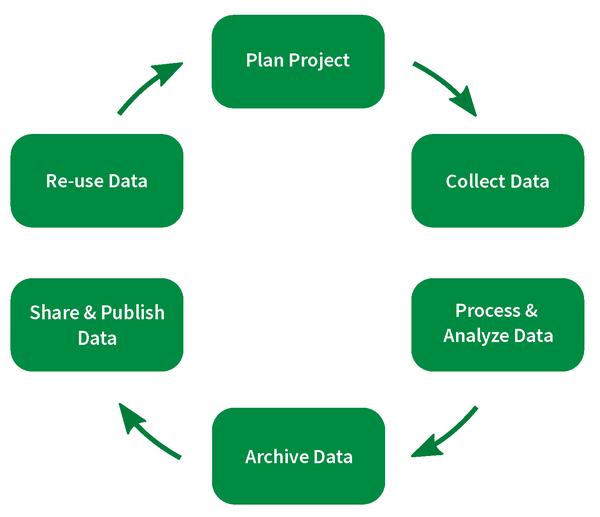
Good Reseacrh Data Management is useful for each scientist / person working in research.
About 60 % of PhD working time is spent with data wrangling (e.g., finding files and harmonizing IDs or formats), this can be reduced with best practice RDM.
Good RDM helps to find your own data faster and reduces both stress and the risk of data loss. It also helps those we collaborate with now or in the future (which might be we ourselves) to understand the content, genesis, interpretation and limitations of a given dataset.
Making (meta-) data findable also helps to reward output that is not put into a high-ranking journal article, it builds your visibility and citability as well as reputation.
See also benefits of complying with
.Research Data Management (RDM)
Research data is a valuable resource. To use it at it's best, good care has to be taken throughout the data lifecycle to ensure high quality and usefulness.
The data lifecycle comprises the following stages (see figure) and implies the following considerations:
1. Project planning
- Which data do you need?
- source, sample size, replicates, type, conditions, time frame, physical sample + information of storage location, etc.
- Is there preexisting data?
- source, owners, format, references, harmonization
- Which metadata describes your data?
- collect as much information as possible at all stages of the lifecycle
- How do you organize your data and which resources or information do you need?
- naming conventions, units, storage, tools, etc.
- How will you care for the data?
- data management plan (DMP), formatting, ownership, access control
- Define schemes and responsibilities.
- names, metadata, etc.
- Plan your required computation capacity.
- especially required RAM
2. Data collection, processing, and analysis
- Do documentation in ELN, record methods, codes.
- (incl. version, settings, parameters)
- Create tools and data directories.
- Ensure storage and backup regimes.
- capacity, local workflows or not, storage time frame, backup schedule and distribution
- deletion criteria
- archiving criteria (when to archive, online or not)
- Collect and store metadata.
- Control your data versions.
- Ensure method stewardship.
- software maintenance, workflow development
- Implement access control and security.
- Update your DMP.
- Write READMEs.
- documenting schemes, variables, (foreign) data sources, methods, links (files, ELN)
- Ensure reproducibility (especially with full documentation) and interoperability.
- check data formats: proprietary software will change formats and render data un-readable after updates
- choose open, free, persistent formats like pdf, csv, txt, rtf
- note differences between operating system especially when working with in-house code)
- if necessary, store software and code together with data (see also )
- Ensure data interpretation follows GSP.
3. Archiving, sharing, publishing, and re-use
- Which data shall be shared / published / archived and where?
- Embargo
- Access level (open / limited / closed)
- Repository
- DOI
- Who pays what?
- Will you publish negative results (as data set / with narrative)?
FAIR stands for findable, accessible, interoperable, and re-usable data [1].
These principles are the common basis for using other’s data and services and making your data available to allow for interdisciplinary and international data-driven research.
But also you will profit yourself: it is easier to understand your own data after a break and your data will be of higher quality.
The following paragraphs give details of the four principles [2] and also of the benefits:
Findable
Precondition
- Independently by machines and humans
- (Meta-) Data contain a globally unique and permanent ID (PID) for long-term citability
- Data are described by comprehensive metadata including provenance
- Metadata contain clearly and explicitly the PID of the data it describes
- (Meta-) Data are registered or indexed in a searchable resource
Accessible
Precondition
- Under well defined conditions
- (Meta-) Data can be retrieved using the PID and a standardized communication protocol
- The protocol is open, free, and universally implementable
- The protocol allows to implement methods of authentication and authorization
- Metadata can be accessed even if data are not (longer) available
Interoperable
Precondition
- Independently by machines and humans
- (Meta-) Data use a formal, accessible, common and publicly applicable language for knowledge representation
- Allows combination with other data sets
- (Meta-) Data use vocabularies that follow FAIR principles
- Metadata contain qualified references to other (meta-) data
- Data formats are non-proprietary, i.e., open, free, and commonly standardized
Re-usable
Objective
- Under properly defined conditions and properly cited
- (Meta-) Data are comprehensively described with a plethora of exact and relevant attributes
- (Meta-) data are published with a defined and accessible data usage license
- (Meta-) data contain detailed information of their genesis and provenance
- (Meta-) data accord domain- relevant community standards
Benefits of FAIRness
- Data quality increases and helps building reputation
- Time efficiency: finding and understanding your data faster, easing hand-overs
- Research results become more accountable if data are published alongside narratives
- Rigorous documentation improves transparency and reproducibility
- Visibility increases due to easier discovery and access of/to datasets, which allows for feedback from colleagues
- Research becomes more efficient: minimizes redundant work
- New research questions arise from previous discoveries and the according dataset
- Collaboration is facilitated, both within a research project as well as world-wide
Open Science is implied when using tax money: our results belong to society. However, not all data can or should be made directly open to everyone (e.g., when a patent shall be issued). To satisfy both needs, a compromise can be to provide open metadata and restricted access to data (access upon request) or to not provide access except for metadata (closed data). FAIR principles guide RDM best practices conforming good GSP, while open science is another ideal that is easily achieved when following FAIR principles.
[1] Wilkinson, M. D., et al.The FAIR Guiding Principles for scientific data management and stewardship. Sci. Data 3:160018 doi: 10.1038/sdata.2016.18 (2016).
[2] GO FAIR: Fair principles [https://www.go-fair.org/fair-principles/] (last accessed 2021-01-27)
At each step of the life cycle, awareness of the
will help to produce reliable and reproducible data and metadata (information & knowledge) as basis for future research and collaboration.RDM begins with creating and naming a folder, naming files, and recording their genesis (e.g., with a link to the corresponding ELN entry).
It involves thinking about and defining requirements and responsibilities when caring for data and results. Ensuring a safe storage and backup strategy (to be discussed / communicated with IT) together with clear ways to find and access data is part of Best Practices.
Taking good care of your own data is data stewardship (the process and attitude good RDM is based on) — therefore it is your responsibility. To support you, the FLI employs a Data Steward, to which you can address all questions and who provides training and consultation regarding all topics around RDM.
- forschungsdaten.info [ , ]
- Kontaktstelle Forschungsdatenmanagement der Friedrich-Schiller-Universität (KSFDM FSU) [ , ]
- Thüringer Kompetenznetzwerk Forschungsdatenmanagement (TKFDM) [ , ]
- MANTRA — Research Data Management Training [ ]
- H2020 Programme — Guidelines on FAIR Data Management in Horizon 2020 (2016) [ ]
- DFG — Leitlinien zum Umgang mit Forschungsdaten (2015) [ ]
- Leibniz Leitlinie zum Umgang mit Forschungsdaten (2018) [ ]